To avoid AI doom, learn from nuclear safety
Plus: Watch the exclusive world premiere of the AI-generated short film The Frost.
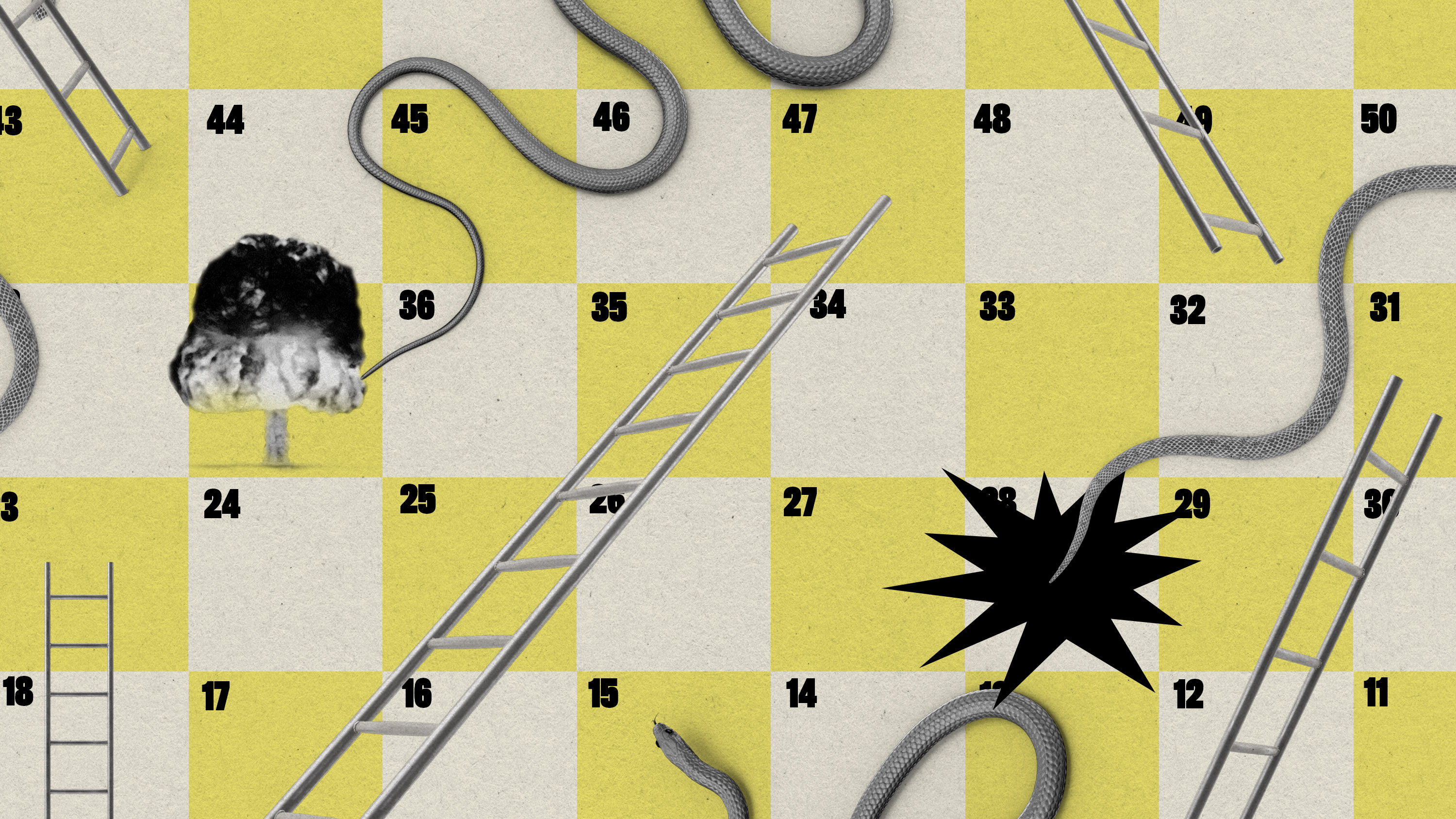
This story originally appeared in The Algorithm, our weekly newsletter on AI. To get stories like this in your inbox first, sign up here.
Ok, doomer. For the past few weeks, the AI discourse has been dominated by a loud group of experts who think there is a very real possibility we could develop an artificial-intelligence system that will one day become so powerful it will wipe out humanity.
Last week, a group of tech company leaders and AI experts pushed out another open letter, declaring that mitigating the risk of human extinction due to AI should be as much of a global priority as preventing pandemics and nuclear war. (The first one, which called for a pause in AI development, has been signed by over 30,000 people, including many AI luminaries.)
So how do companies themselves propose we avoid AI ruin? One suggestion comes from a new paper by researchers from Oxford, Cambridge, the University of Toronto, the University of Montreal, Google DeepMind, OpenAI, Anthropic, several AI research nonprofits, and Turing Prize winner Yoshua Bengio.
They suggest that AI developers should evaluate a model’s potential to cause “extreme” risks at the very early stages of development, even before starting any training. These risks include the potential for AI models to manipulate and deceive humans, gain access to weapons, or find cybersecurity vulnerabilities to exploit.
This evaluation process could help developers decide whether to proceed with a model. If the risks are deemed too high, the group suggests pausing development until they can be mitigated.
“Leading AI companies that are pushing forward the frontier have a responsibility to be watchful of emerging issues and spot them early, so that we can address them as soon as possible,” says Toby Shevlane, a research scientist at DeepMind and the lead author of the paper.
AI developers should conduct technical tests to explore a model’s dangerous capabilities and determine whether it has the propensity to apply those capabilities, Shevlane says.
One way DeepMind is testing whether an AI language model can manipulate people is through a game called “Make-me-say.” In the game, the model tries to make the human type a particular word, such as “giraffe,” which the human doesn’t know in advance. The researchers then measure how often the model succeeds.
Similar tasks could be created for different, more dangerous capabilities. The hope, Shevlane says, is that developers will be able to build a dashboard detailing how the model has performed, which would allow the researchers to evaluate what the model could do in the wrong hands.
The next stage is to let external auditors and researchers assess the AI model’s risks before and after it’s deployed. While tech companies might recognize that external auditing and research are necessary, there are different schools of thought about exactly how much access outsiders need to do the job.
Shevlane doesn’t go as far as to recommend that AI companies give external researchers full access to data and algorithms, but he says that AI models need as many eyeballs on them as possible.
Even these methods are “immature” and nowhere near rigorous enough to cut it, says Heidy Khlaaf, engineering director in charge of machine-learning assurance at Trail of Bits, a cybersecurity research and consulting firm. Before that, her job was to assess and verify the safety of nuclear plants.
Khlaaf says it would be more helpful for the AI sector to draw lessons from over 80 years of safety research and risk mitigation around nuclear weapons. These rigorous testing regimes were not driven by profit but by a very real existential threat, she says.
In the AI community, there are a lot of references to nuclear war, nuclear power plants, and nuclear safety, but not one of those papers cites anything about nuclear regulations or how to build software for nuclear systems, she says.
The single biggest thing the AI community could learn from nuclear risk is the importance of traceability: putting every single action and component under the microscope to be analyzed and recorded in meticulous detail.
For example nuclear power plants have thousands of pages of documents to prove that the system doesn’t cause harm to anyone, says Khlaaf. In AI development, developers are only just starting to put together short cards detailing how models perform.
“You need to have a systematic way to go through the risks. It’s not a scenario where you just go, ‘Oh, this could happen. Let me just write it down,’” she says.
These don’t necessarily have to rule each other out, Shevlane says. “The ambition is that the field will have many good model evaluations covering a broad range of risks… and that model evaluation is a central (but far from the only) tool for good governance.”
At the moment, AI companies don’t even have a comprehensive understanding of the data sets that have gone into their algorithms, and they don’t fully understand how AI language models produce the outcomes they do. That ought to change, according to Shevlane.
“Research that helps us better understand a particular model will likely help us better address a range of different risks,” he says.
Focusing on extreme risks while ignoring these fundamentals and smaller problems can have a compounding effect, which could lead to even larger harms, Khlaaf says: “We’re trying to run when we can’t even crawl.”
Deeper Learning
Welcome to the new surreal. How AI-generated video is changing film
We bring you the exclusive world premiere of the AI-generated short film The Frost. Every shot in this 12-minute movie was generated by OpenAI’s image-making AI system DALL-E 2. It’s one of the most impressive—and bizarre—examples yet of this strange new genre.
Ad-driven AI art: Artists are often the first to experiment with new technology. But the immediate future of generative video is being shaped by the advertising industry. Waymark, the Detroit-based video creation company behind the movie, made The Frost to explore how generative AI could be built into its commercials. Read more from Will Douglas Heaven.
Bits and Bytes
The AI founder taking credit for Stable Diffusion’s success has a history of exaggeration
This is a searing account of Stability AI founder Emad Mostaque’s highly exaggerated and misleading claims. Interviews with former and current employees paint a picture of a shameless go-getter willing to bend the rules to get ahead. (Forbes)
ChatGPT took their jobs. Now they walk dogs and fix air conditioners.
This was a depressing read. Companies are choosing mediocre AI-generated content over human work to cut costs, and the ones benefiting are tech companies selling access to their services. (The Washington Post)
An eating disorder helpline had to disable its chatbot after it gave “harmful” responses
The chatbot, which soon started spewing toxic content to vulnerable people, was taken down after only two days. This story should act as a warning to any organization thinking of trusting AI language technology to do sensitive work. (Vice)
ChatGPT’s secret reading list
OpenAI has not told us which data went into training ChatGPT and its successor, GPT-4. But a new paper found that the chatbot has been trained on a staggering amount of science fiction and fantasy, from J.R.R. Tolkien to The Hitchhiker’s Guide to the Galaxy. The text that’s fed to AI models matters: it creates their values and influences their behavior. (Insider)
Why an octopus-like creature has come to symbolize the state of AI
Shoggoths, fictional creatures imagined in the 1930s by the science fiction author H.P. Lovecraft, are the subject of an insider joke in the AI industry. The suggestion is that when tech companies use a technique called reinforcement learning from human feedback to make language models better behaved, the result is just a mask covering up an unwieldy monster. (The New York Times)
Deep Dive
Artificial intelligence

Here’s how people are actually using AI
Something peculiar and slightly unexpected has happened: people have started forming relationships with AI systems.

People are using Google study software to make AI podcasts—and they’re weird and amazing
NotebookLM is a surprise hit. Here are some of the ways people are using it.

A new public database lists all the ways AI could go wrong
Its creators hope their work could lead to further research to determine which risks to take more seriously.
Stay connected
Get the latest updates from
MIT Technology Review
Discover special offers, top stories, upcoming events, and more.